Barriers To Data Driven Web Optimization
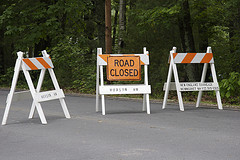
Photo: StarrGazr
One of the greatest advantages of online marketing is the marketers’ ability to quickly adjust and respond to the data they can obtain directly from their interactions with their market. However, each organization is at a different stage in terms of their online measurement sophistication and each faces barriers to becoming more effective at optimizing their web marketing.
Avinash Kaushik recently posted a good blog entry on Barriers To An Effective Web Measurement Strategy. In the blog, he recommends some first steps in overcoming the following 11 barriers to an effective online measurement strategy that were cited in Econsultancy’s Online Measurement and Strategy Report.
1. Lack of budget/resources (45%)
2. Lack of strategy (31%)
3. Siloed organization (29%)
4. Lack of understanding (25%)
5. Too much data (18%)
6. Lack of senior management buy-in (18%)
7. Difficulty reconciling data (17%)
8. IT blockages (17%)
9. Lack of trust in analytics (16%)
10. Finding staff (12%)
11. Poor technology (9%)
While I did find Avinash’s insights on each of the barriers valuable, I think the list of barriers is flawed in that it confuses the symptoms with the actual barriers. Could you imagine, for example, if a baseball manager explained that the reason his team was losing was because he was getting “too much data†from his statistician (point 5 from the barrier list). If he did, he would not be manager for long. It’s the manager’s job to determine which stats are important and will make a difference in his game strategy. While I’m sure there are many executives and analysts who feel that they do have to sift through too much data, the true barrier is the lack of a good strategy that focuses the organization on a few core metrics that get to the heart of what you are trying to accomplish.
Given our experience in helping many organizations with their data driven optimization, I think the 11 point barrier list could be refined down to the following 4 core barriers.
1. Strategy
It all starts with a good strategy. It’s necessary for obtaining management buy-in and for ultimately attaining the ROI on any investments made in optimization initiatives. It is critical that the strategy identify a small set of critical metrics that are meaningful to management and drive any ROI business case.
It’s also important to recognize that the web optimization strategy needs to evolve as the web optimization capabilities of the organization evolve. The strategy is about pinpointing the most important constraints that you need to overcome and focusing your time and resources on making that happen. Often this may require small steps first to gain credibility and management buy-in.
2. Management Buy-in
Not surprisingly, once you obtain management buy-in, many of the symptoms begin to disappear. Certainly lack of budget/resources and IT blockages begin to dissipate if you have the support of senior management. While it is likely the most important barrier to overcome, this can often be the most difficult. It’s also important to recognize that until management is willing to make some level of investment in analytics and optimization, then they have not really bought-in.
This often creates a chicken or the egg situation where the online marketer asks, “How do I credibly demonstrate ROI on the investment prior to making the investment�  Early on this is where the hard work and innovative thinking must occur to implement examples of how data-driven initiatives can provide the ROI necessary to develop a strong business case. The good news is that with the use of inexpensive, often free, tools in combination with some good analysis, it is not too difficult to gain credibility by demonstrating substantive ROI. This is often the key objective of organizations that are relatively early in their web optimization evolution.
3. Infrastructure for Testing, Analysis, and Change
The infrastructure I am addressing here includes the website or ecommerce management technology as well as the implementation of the analytics tools. We have come across many situations where online marketers have properly identified what are potentially significant improvements that will really move the needle on their core metrics, but the amount of work involved to test or implement the changes were believed to be too significant to justify the investment.
This can be a daunting challenge in that the investment in the current infrastructure and the staff to support it may have been significant and modifications may be difficult and expensive. In many cases this requires working actively with someone who is technically proficient enough to implement some relatively small tests across several areas that when viewed together make a strong case for an investment in a new infrastructure or new component to that infrastructure. In other cases, this is more of an uphill battle and the key becomes just recognizing the constraints and focusing on those areas where improvements can be made.
4. Skills and Time for Testing, Analysis, and Change
In my opinion this is the most overlooked and most underestimated barrier that must be overcome.  Many organizations that have implemented analytics either largely ignore the analytics reports, don’t have time to analyze them, or generate reports that provide little in terms of insights that stimulate changes that improve the critical metrics and provide a high ROI. In order for an organization to effectively implement data-driven optimization, they must have time from the personnel who know what data they should be focused on, what tests should be implemented, and what actions should be taken as a result of the analysis. This, by the way, does not mean that an analyst should be doing all of the analysis. In fact, quite the opposite, they need to know how to get the appropriate people throughout the organization involved in the analysis (the “Why†behind the “What†happened).  As the benefits of this time become apparent, it becomes easier to build this testing and analysis into normal work processes.
Most organizations also tend to under invest in the time required from the personnel who are necessary to effectively carry out tests. For example, designing multiple versions of a page for A/B or multivariate testing can pay significant dividends. Organizations need to plan accordingly for copywriting and design resources in order to make these tests successful.
By focusing in on and addressing these four key areas, the other issues on the Econsultancy list will likely be addressed in the process. What do you think of the 11 barriers identified in the Econsultancy study?